In Part 1, we explored the concept of Functional Mock-up Units (FMUs) and how they enable simulation models to operate across different software environments. As engineering workflows become more complex and tool chains more diverse, FMUs offer a compelling solution: streamlining integration and accelerating development.
In this follow-up, we turn our attention to a key application area where FMUs are proving especially valuable: battery modeling. From battery-powered devices in need for Digital-Twins to Energy Management Systems, the demand for accurate, flexible, and fast battery models has never been higher. FMUs provide a practical way to meet that demand, and BattGenie’s battery FMU delivers a solution built for today’s electrification challenges.
Recap – Why Battery FMUs Matter:
Before diving into the specifics of BattGenie’s battery FMU, it’s worth revisiting what makes FMUs so valuable in battery simulation in the first place.
Battery FMUs combine high-fidelity models with tool-agnostic deployment, allowing engineers to simulate real-world battery behavior across different systems—without needing to rebuild the model for each platform. This portability makes it easier to integrate battery dynamics into broader system simulations, whether for device design, energy management strategies, or early-stage BMS development.
Battery FMU Advantages – A Quick Recap
- System Integration: Battery FMUs can be easily added to larger simulations—like electric vehicle powertrains or grid storage networks—without major rework.
- Cross-Platform Flexibility: Whether you’re using MATLAB, Modelica-based platforms, or Python, an FMU keeps the core physics intact, ensuring reliable results across different environments.
- Scalability: Battery FMUs can handle everything from simple equivalent circuit models to detailed electrochemical or thermal simulations.
- Modular & Easy to Update: FMUs make it simple to swap or refine battery models without disrupting entire simulations.
- Faster Development & Testing: Instead of spending time re-implementing models, teams can focus on analysis, optimization, and getting battery-powered solutions to market faster.
Introducing BattGenie’s Battery FMU:
BattGenie’s battery FMU brings together advanced battery modeling and practical deployment. Designed to be both physics-informed and tool-agnostic, this FMU captures the real-world behavior of lithium-ion batteries while remaining flexible enough to integrate into a wide variety of simulation workflows.
At its core, the BattGenie FMU enables engineers and researchers to simulate how batteries perform under realistic loading conditions, thermal environments, and usage patterns. Whether used for system-level analysis, battery management systems (BMS) testing, or component optimization, the model is purpose-built for modern electrification challenges.
Unlike many black-box or table-based models, the BattGenie FMU emphasizes speed, transparency, fidelity, and ease of use. It provides just the right balance of computational efficiency and physical accuracy—making it a reliable building block for both early-stage design and validation and integration phases.
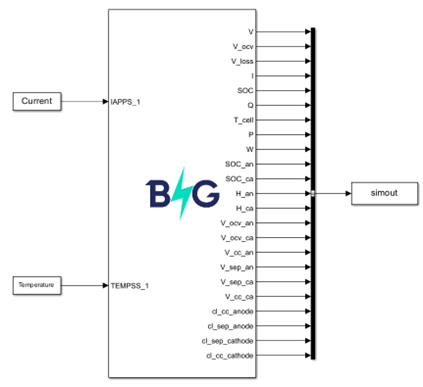
Key Features & What Sets It Apart:
BattGenie’s battery FMU stands out by blending high-fidelity physics with a user-friendly, platform-flexible design. While many battery models are either oversimplified or require heavy customization to use across tools, BattGenie’s FMU is ready to deploy, adapt, and scale—without compromising accuracy or ease of integration. One FMU, many form factors.
Key features:
- Multi-Physics Modeling: The FMU doesn’t stop at electrochemical behavior. It incorporates thermal dynamics, enabling more comprehensive simulations of battery performance over time and under varying environmental conditions.
- Speed: Engineered for performance, the FMU runs faster than state-of-the-art models, even when simulating complex interactions. Its compact design supports parallel execution on multiple cores or compute nodes, enabling high-throughput simulations across diverse scenarios.
- Parameter-agnostic: The FMU performs reliably across a wide range of cell characteristics, minimizing the rework needed when switching between chemistries, capacities, or internal resistance profiles.
- Modular & Scalable: From single-cell models to full battery packs, the FMU’s architecture scales effortlessly. Its modular design supports system-level integration across different pack sizes and simulation needs.
- Transparent: Unlike black-box models, BattGenie’s FMU is interpretable and adaptable, offering insight into the parameters and behaviors being simulated. This transparency is critical for teams working on performance optimization, safety validation, and long-term reliability analysis.
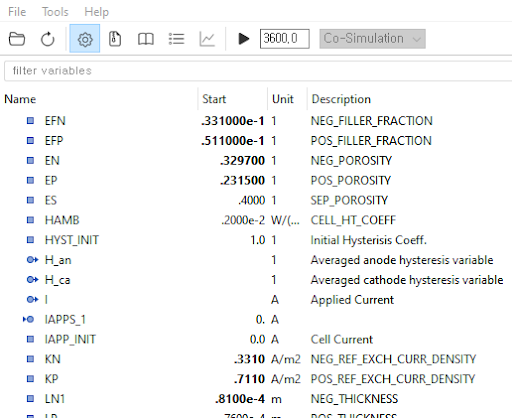
The BattGenie FMU runs in co-simulation mode, using our internally developed numerical solver optimized for performance. This ensures that the model executes efficiently without depending on external solvers.
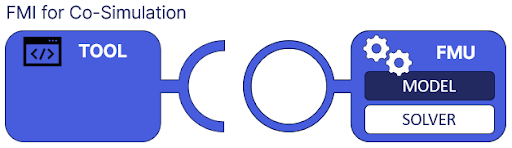
Why BattGenie’s FMU Delivers More? Answer: Traditional battery models like lookup tables or low-order equivalent circuit models often fall short when it comes to accuracy, scalability, or integration. Proprietary simulation blocks, while sometimes more sophisticated, can limit flexibility by locking teams into specific software ecosystems or requiring intensive customization. BattGenie’s FMU takes a more open, scalable approach. With physics-based modeling, FMI compliance, and broad tool compatibility, it delivers consistent, high-fidelity performance across platforms without the overhead. The result is a model that is not only more accurate and flexible, but also more collaborative, portable, and ready for deployment in real-world engineering workflows.
Simulation-Ready in Any Environment:
BattGenie’s battery FMU is designed with versatility in mind. Because it adheres to the Functional Mock-up Interface (FMI) standard, it can be used seamlessly across a wide range of simulation tools without any need for reimplementation or translation. Build once, simulate anywhere.
This cross-platform compatibility means teams can collaborate using the tools they know best, while still working with a consistent, high-fidelity battery model.
BattGenie’s FMU supports integration with:
- MATLAB/Simulink – Ideal for state estimation & control design, model-based development, and system simulation
- Python environments – Via libraries such as FMPy, making it suitable for custom workflows or scripting
- Other tools – Includes Modelica environments and C/C++ based simulators commonly used for multi-domain modeling, embedded systems, or real-time applications.
The model is packaged using the FMI 3.0 standard, the latest version of the FMU specification. This allows for more robust handling of input/output dependencies, improved variable initialization, and better co-simulation support across modern simulation tools.
Where It’s Headed ? Answer: BattGenie’s FMU is built to support a variety of use cases—from EV powertrain simulations and grid storage modeling to aerospace systems and battery R&D. Its flexibility makes it well-suited for applications that demand accuracy, speed, and easy integration across complex systems.
Looking Ahead
Battery FMUs are more than just a convenient way to move models between tools—they represent a shift toward more collaborative, efficient, and scalable engineering. BattGenie’s FMU is designed with this future in mind: flexible to adapt, robust to avoid failure, extremely fast and accurate to trust, and ready to meet the demands of modern electrification challenges.
In the next installment of this series, we’ll go deeper into the technical structure and modeling approach behind the BattGenie FMU. We’ll explore how the model is implemented in MATLAB, walk through the core physics behind its behavior, and share insights on parameterization, key inputs, and validation metrics.
Stay tuned for Part 3, where we’ll pull back the curtain and show exactly how the FMU works under the hood.
Venkat Subramanian
CTO, Chief Scientific Advisor, and Co-Founder
Prof. Venkat Subramanian is currently the Ernest Dashiell Cockrell II Professor of Mechanical & Material Science Engineering at the University of Texas, Austin.
His research interests include energy systems engineering, electrochemical engineering, computationally efficient algorithms for state-of-charge (SOC) and state-of-health (SOH) estimation of lithium-ion batteries, multiscale simulation, and design of energetic materials, kinetic Monte Carlo methods, model-based battery management system for electric transportation, and renewable microgrids and nonlinear model predictive control. Prof. Subramanian was awarded the Dean’s award for excellence in graduate study in 2001 for his doctoral research.
He is a Fellow of the Electrochemical Society and a past Technical Editor of the Journal of the Electrochemical Society. He was also the chair of the IEEE Division of the Electrochemical Society. His codes for Lithium-ion batteries are the fastest reported in the literature and his algorithm for solving index 1 nonlinear DAEs is the most robust compared to any other algorithm reported as of today.
Prof. Subramanian received his B.Tech. degree in Chemical and Electrochemical Engineering from the Central Electrochemical Research Institute (CECRI), Karaikudi, India, in 1997 and the Ph.D. degree in Chemical Engineering from the University of South Carolina, Columbia, SC, USA, in 2001.
Manan Pathak
CEO and Co-Founder
Dr. Manan Pathak is the Chief Executive Officer and co-founder of BattGenie.
He earned his PhD at the University of Washington, where he obtained his graduate thesis on model-based Battery Management Systems. He has 7+ peer-reviewed publications with over 300 citations, and extensive experience with physics-based battery models, numerical methods and derivation of optimal charging profiles.
Chintan Pathak
CPO and Co-Founder
Dr. Chintan Pathak is the Chief Product Officer and co-founder of BattGenie.
He earned his PhD from the University of Washington and he obtained his graduate thesis on optimal locations of battery charging stations in the state of Washington. He has over 13 years of experience in software engineering and embedded systems.
Akshay Subramaniam
Battery Modeling Scientist
Akshay Subramaniam leads electrochemical model development and identification tasks at BattGenie. He also contributes towards BMS algorithm development and validation, and helps maintain our models, databases, and testing pipelines. He received his Ph.D. from the University of Washington during which he gained extensive experience in the development of control-oriented electrochemical models. He has 10+ peer-reviewed publications and is proficient in several aspects of battery systems engineering including numerical simulation techniques, optimization for design and fast charging, parameter estimation, and battery data analysis.
Taejin Jang
Battery Simulation Scientist
Dr. Taejin Jang is a Battery Simulation Scientist at BattGenie. Dr. Jang received his Ph.D in Materials Science from University of Texas at Austin and an MS in Chemical Engineering from UW. He also has BS and MS degrees in Materials Science & Chemical Engineering from Yokohama National University in Japan. He spent three years in the automotive devices industry at Samsung Electronics. He has 7+ years’ experience in battery modeling and simulation, encompassing Li-ion and next-generation batteries.
Bing Syuan Wang
Senior Battery Software and Data Engineer
Bing Syuan Wang is the Senior Battery Software and Data Engineer at BattGenie.
He earned his Masters in Electrical Engineering from the University of Washington. He has over 6 years’ experience in software engineering and in working with battery data.
Aditya Parsai
Fullstack Software Engineer
Aditya Parsai is a Fullstack Software Engineer at BattGenie. He graduated in Civil Engineering from IIT(BHU). With 8 years’ experience, he contributes to helping businesses succeed in the digital space by staying attuned to the evolving tech landscape. His work spans from front-end development to back-end system engineering, ensuring smooth integration and functionality. He recognizes the importance of storytelling and is adept in translating complex ideas into user-friendly interfaces to enhance user experiences.